In the rapidly evolving landscape of technology, artificial intelligence (AI) and machine learning have emerged as transformative forces, reshaping industries and redefining possibilities. Yet, behind the curtain of these groundbreaking advancements lies an often-overlooked hero: data annotation. As AI continues to permeate our daily lives, a critical question surfaces: Is data annotation tech legit? This comprehensive exploration delves into the world of data annotation, examining its legitimacy, impact, and future role in our AI-driven society.
What Exactly Is Data Annotation Tech?
At its core, data annotation is the process of labeling raw data to make it intelligible and usable for machine learning models. It’s akin to teaching a computer to perceive and interpret the world through a human lens. Annotators engage in various tasks, including tagging images, transcribing audio, labeling text, and classifying video content. This meticulous work creates a crucial bridge between raw information and AI comprehension.
The realm of data annotation encompasses several key types:
- Image annotation: This involves drawing bounding boxes around objects, segmenting images, or labeling specific features within pictures.
- Text annotation: Tasks include sentiment analysis, entity recognition, and content classification.
- Audio annotation: Primarily focuses on transcription and emotion labeling in speech.
- Video annotation: Encompasses object tracking, action recognition, and scene description.
Real-world example: Consider the development of autonomous vehicles. These technological marvels rely heavily on annotated images and video to recognize and respond to pedestrians, traffic signs, other vehicles, and countless other elements on the road. Without the foundation of data annotation, these AI-driven cars would essentially be blind to their surroundings, rendering them both useless and dangerous.
Read This Post: What is a Tech Truck Roll?
The Legitimacy Question: Why It Matters
The question of whether data annotation tech is legitimate isn’t merely an academic exercise. It’s an inquiry with profound implications for AI development, ethics, and the global economy.
- Impact on AI development: High-quality annotated data serves as the bedrock for accurate AI models. The age-old programming adage “garbage in, garbage out” applies here with particular relevance. The quality and accuracy of annotated data directly influence the performance and reliability of AI systems.
- Ethical considerations: The process of data annotation raises important ethical questions. Who determines what gets labeled and how? Bias in annotation can lead to biased AI systems, potentially perpetuating or even exacerbating societal inequalities. For instance, if facial recognition systems are trained on datasets that underrepresent certain ethnic groups, it could lead to higher error rates and discriminatory outcomes for those groups.
- Economic implications: The data annotation market is experiencing explosive growth, creating new job opportunities but also raising questions about labor practices and the long-term impact of automation in this field.
The Case for Data Annotation Tech
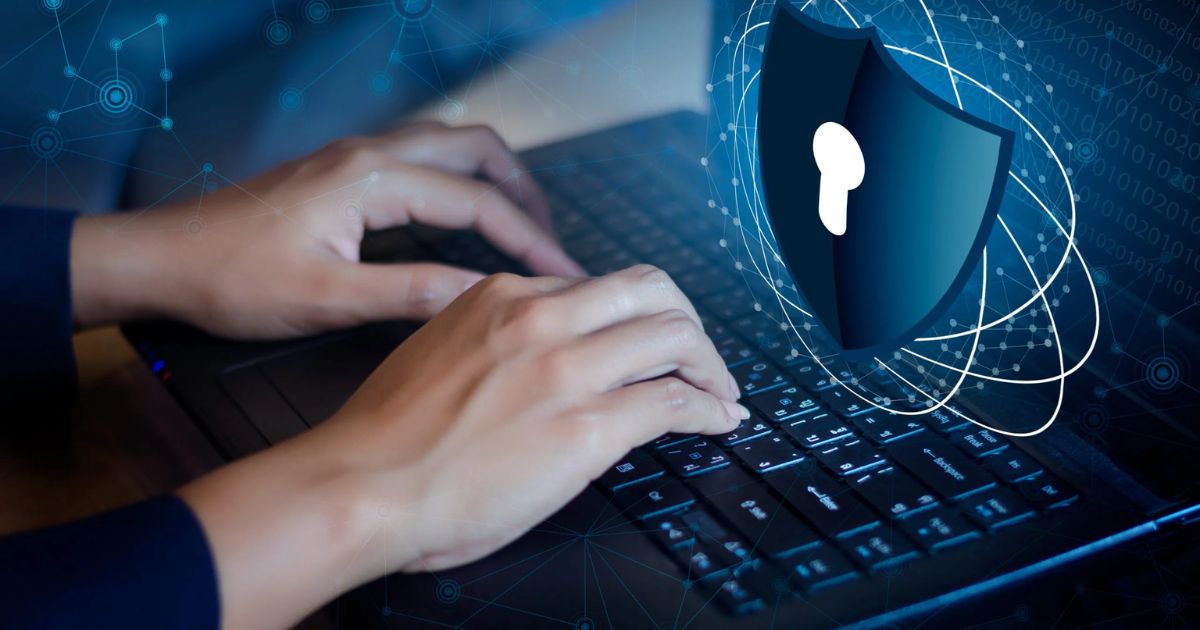
Fueling AI Advancements
Data annotation is the critical ingredient powering numerous breakthroughs in AI. Its impact is far-reaching, enabling:
- More sophisticated image recognition systems capable of identifying objects, faces, and scenes with unprecedented accuracy.
- Natural language processing models that can understand context, nuance, and even sarcasm in human communication.
- Predictive models with the capability to forecast everything from weather patterns and natural disasters to stock prices and consumer behavior.
Industry Applications
The applications of data annotation technology span across various industries, revolutionizing processes and opening new possibilities:
Industry | Application | Impact |
Healthcare | Diagnostic imaging | Earlier and more accurate detection of diseases |
Autonomous vehicles | Road scene understanding | Safer and more efficient transportation |
E-commerce | Product recommendations | Highly personalized shopping experiences |
Agriculture | Crop and soil analysis | Optimized farming practices and increased yields |
Finance | Fraud detection | Enhanced security and risk management |
Case study: Healthcare Breakthrough A landmark study published in Nature Medicine demonstrated the power of well-annotated data in healthcare. Researchers developed an AI system trained on meticulously annotated mammograms. The results were astounding: the system could detect breast cancer with accuracy comparable to, and in some cases exceeding, that of human radiologists. This technology holds the potential to revolutionize early cancer detection, potentially saving countless lives through earlier and more accurate diagnoses.
Job Creation and Economic Growth
The data annotation market is experiencing explosive growth. According to a report by Grand View Research, the global data annotation tools market is expected to reach $3.5 billion by 2028, growing at a compound annual growth rate (CAGR) of 27.1% from 2021 to 2028. This surge is creating a wealth of job opportunities, particularly in the realm of remote work.
Notably, this growth is having a significant impact in developing countries, where data annotation jobs are providing new avenues for employment and economic advancement.
“Data annotation is not just about technology; it’s about empowering people to contribute to the AI revolution. It’s creating opportunities for individuals around the world to participate in the digital economy, regardless of their location,” says Maria Rodriguez, CEO of Global Annotation Solutions.
Challenges and Criticisms
Despite its immense promise, data annotation technology faces several significant challenges and criticisms that must be addressed.
Quality Control Issues
One of the primary concerns in data annotation is the potential for human error. Annotators, despite their best efforts, can make mistakes or introduce inconsistencies into datasets. These errors, when propagated through AI models, can lead to flawed or unreliable systems.
Moreover, annotator bias presents a serious challenge. If annotators consistently mislabel or misinterpret certain types of data – for instance, consistently mislabeling certain ethnic groups in image datasets – it can lead to AI systems that perpetuate or amplify discriminatory outcomes.
Privacy Concerns
Data annotation often involves handling vast amounts of data, some of which may be sensitive or personal in nature. This raises significant privacy concerns:
- Data breaches: There’s always a risk that annotated datasets could be compromised, leading to the exposure of personal information.
- Misuse of personal data: Without proper safeguards, there’s potential for annotated data to be used for purposes beyond its original intent, raising ethical and legal questions.
Companies engaged in data annotation must implement robust security measures and adhere to strict data protection protocols to mitigate these risks.
Ethical Dilemmas
The field of data annotation is not immune to ethical challenges:
- Low wages: Many data annotation jobs, particularly those outsourced to developing countries, are low-paid. This raises concerns about exploitation and fair labor practices in the global AI supply chain.
- Job displacement: As annotation processes become increasingly automated, there’s a growing fear that it could lead to job losses in the long run, particularly affecting those who have come to rely on data annotation as a source of income.
The Future of Data Annotation Tech
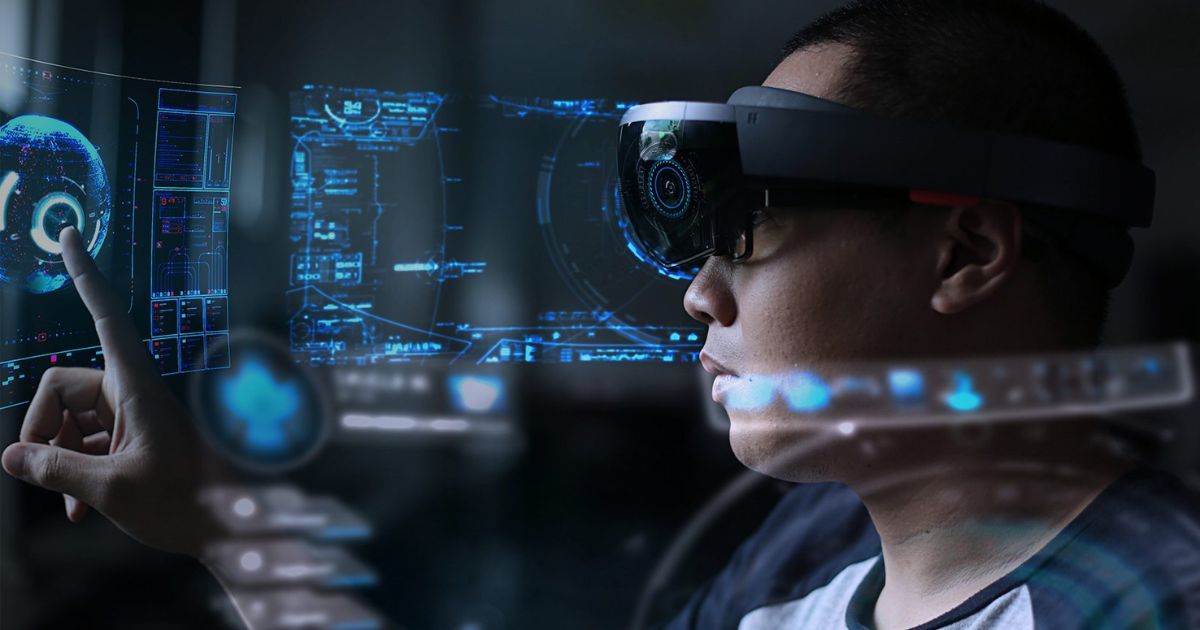
As we continue to grapple with the question “is data annotation tech legit,” it’s crucial to look ahead at emerging trends and potential breakthroughs that could shape the future of this field.
Emerging Trends
- AI-assisted annotation: The future of data annotation likely lies in a hybrid approach, where AI systems pre-annotate data, and humans perform quality checks and refinements. This could significantly speed up the annotation process while maintaining high levels of accuracy.
- Crowdsourcing and gamification: Platforms like Amazon Mechanical Turk are already turning data annotation into a global, gamified effort. This trend is likely to continue, with more sophisticated platforms emerging that make data annotation more engaging and accessible to a wider pool of contributors.
- Specialized annotation tools: As the demand for high-quality annotated data grows, we’re likely to see the development of more sophisticated, industry-specific annotation tools. These could include VR-based annotation systems for 3D data or specialized tools for medical image annotation.
Potential Breakthroughs
- Automated quality control: The development of AI systems that can detect and correct annotation errors in real-time could revolutionize the field, dramatically improving the quality and reliability of annotated datasets.
- Ethical frameworks: The creation of industry-wide standards and ethical frameworks for fair and unbiased data annotation practices could address many of the current concerns about bias and exploitation in the field.
- Transfer learning in annotation: Advancements in transfer learning could allow annotation models trained on one type of data to be quickly adapted for use with different, but related, types of data. This could significantly reduce the time and resources required for annotation in new domains.
Is Data Annotation Tech Really Legit?
After a thorough examination of the evidence, it becomes clear that data annotation technology is not just legitimate, but essential for the continued advancement of AI. However, this legitimacy comes with important caveats and responsibilities.
Pros:
- Enables significant AI advancements across a wide range of industries
- Creates job opportunities, particularly in developing economies
- Improves the accuracy and reliability of AI models
- Facilitates breakthroughs in fields like healthcare and autonomous transportation
Cons:
- Faces ongoing challenges with quality control and consistency
- Raises significant privacy and data security concerns
- Presents ethical dilemmas related to labor practices and potential job displacement
- Can perpetuate or amplify biases if not carefully managed
Expert opinion on the legitimacy of data annotation tech is largely positive, albeit with acknowledgment of its challenges. Dr. Emily Chen, AI researcher at MIT, offers this perspective: “Data annotation is the foundation of modern AI. Without it, we’d be stuck in the dark ages of machine learning. However, we must remain vigilant about addressing its challenges, particularly in terms of bias and ethics.”
Conclusion
So, is data annotation tech legit? The answer is a resounding yes, but with important qualifications. It’s a powerful and necessary tool that’s driving the AI revolution, creating economic opportunities, and enabling technological breakthroughs that have the potential to improve lives across the globe. From healthcare to transportation, from e-commerce to agriculture, the impact of well-annotated data is being felt in virtually every sector of the economy.
However, we must remain vigilant about the challenges posed by data annotation technology. Quality control, ethical considerations, and the potential for bias are ongoing concerns that require constant attention and innovative solutions. As we move forward, it’s crucial for all stakeholders – from tech enthusiasts and industry professionals to policymakers and ethicists – to stay informed and engaged in the conversation surrounding data annotation technology.